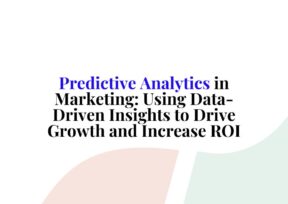
Predictive Analytics in Marketing: Using Data-Driven Insights to Drive Growth and Increase ROI
Last update: 23 February 2024 at 10:12 am
Predictive analysis is a type of advanced analytics that forecasts future events, outcomes and overall behaviour. It employs statistical techniques, including sophisticated predictive modelling and machine learning algorithms, to scrutinize both historical data and current data. It also gauges the likelihood of something happening, even if it isn’t visible on your business radar.
With the data generated through predictive analytics in marketing, you can –
- make better business decisions.
- optimize your marketing strategy.
- understand your target audience.
- target the identified potential leads.
- deliver desired or needed services and products, thereby increasing your revenues and ROI.
With that being said, now let’s dive into this blog to gain an in-depth understanding of how predictive analysis can revolutionize your marketing strategies for good. Let’s begin!
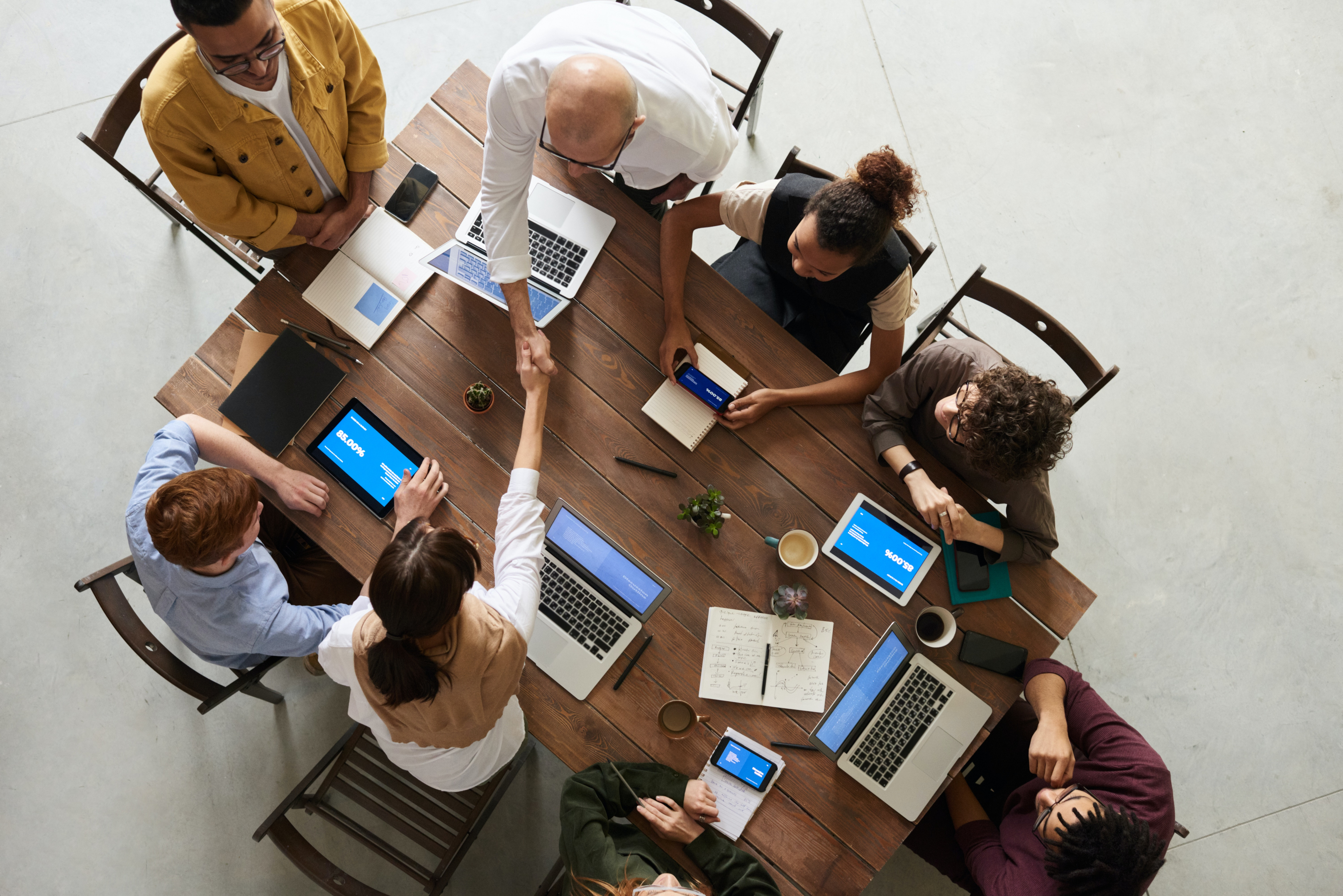
1- Understanding Predictive Analytics
For decades now, marketers have been using data to comprehend and enhance the effectiveness of marketing campaigns. In fact, these marketing efforts have only gotten advanced over the years.
The tech-savvy customers of the modern world are spoilt for choice. They can order what they want whenever they want. And this has led to fierce competition among service providers, vendors and retailers.
Predictive analytics allows marketers to stay ahead of consumer desires and trends by assisting them in comprehending consumer behaviour and predicting future shifts. This allows for effective planning of the campaigns.
Currently, marketers use three key types of predictive analytics model to create effective and dynamic media plans that eventually results in enhanced consumer experience and ROI. These include,
- Cluster models – This analytics model uses algorithms for audience segmentation based on past purchases, demographic data and past brand engagement.
- Propensity models – Here, the consumer’s chances of doing something are assessed, such as converting, disengaging or acting on an offer.
- Recommendation filtering – This analytics model assesses the past purchase history to determine any additional sales opportunities.
Since the application of predictive analytics is extensive, key components are often required for maintaining effectiveness, such as –
1.1- Data sourcing
To develop accurate predictions and produce a decision tree, a data source needs to be vetted thoroughly and capably.
Data sourcing is typically the first step where you will require identifying gaps in the outputs. Also, reviewing the privacy factors and regulatory components of the data can help identify the given source’s validity.
1.2- Data utility
The next step is to determine how useful the available data is.
It is imperative that you gather your own data, especially when it comes to predicting future events instead of relying on third-party data. With your own data, you can be sure of its utility.
1.3- Machine learning, automation and deep learning
When it comes to certain predictive analytics tools, business processes lean towards the use of business intelligence. But, business analysts and data scientists trend towards automated regression techniques, including linear and logistic regression.
|
Discover the most relevant agencies for your project based on your own specific requirements.
Find an agency!This is because of the data regulations in terms of predictive analytics software and marketing tech.
Many vendors are using advanced business analytics and data modeling because it is challenging to regulate the compliance of data automation in real time.
1.4- Objectives and usage
As a user, you must identify whether or not your predictive tools are generating accurate information post-data preparation. Also, you must know if you can correlate to your business’s objectives.
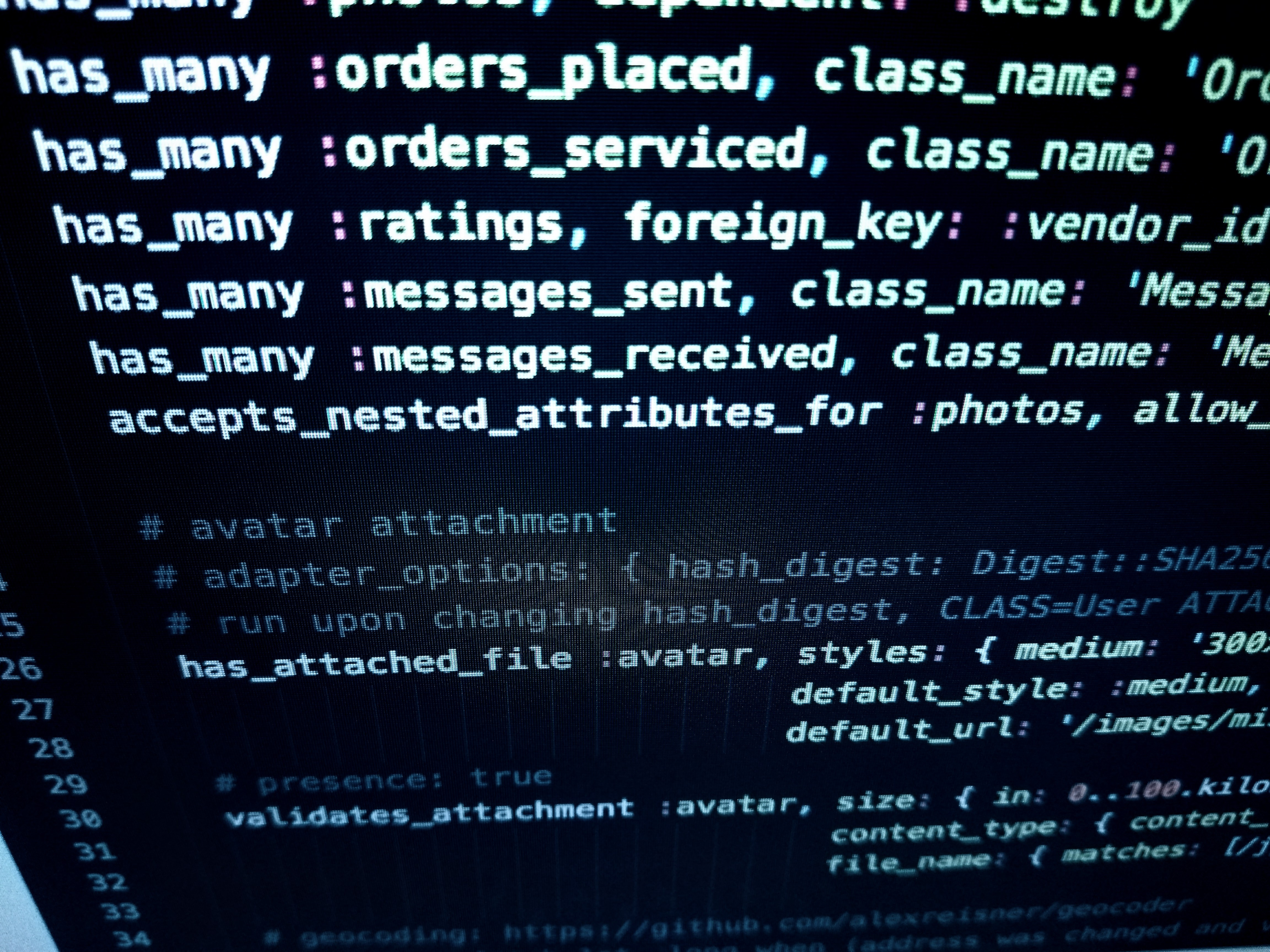
2- Collecting and Preparing Data
Predictive marketing analytics utilizes data and statistical techniques like predictive modelling and machine learning (ML) to predict outcomes.
Through the examination of large amounts of data, professionals can identify trends and behaviours in the industry. And as mentioned earlier, these predictions assists in gaining valuable insights further leads to better-informed investment and business decision.
Hence, for this very reason identifying the sources of all the available relevant data is crucial. Once you have datasets at your disposal, it is time to conduct an exploratory data analysis (EDA).
This helps determine –
- What kind of data do you have?
- The feature the data possess
- The outcome or target variable
- The correlation among the data feature
EDA helps understand the data better and design suitable models.
Certain collected customer data can also feature a lot of redundant data. When such data is fed into the model as input, the chances of the model making the wrong prediction becomes high. With a proper EDA on the data, all the null values, outliers and unnecessary elements are identified and cleaned. Once the patterns in the data are identified, it becomes easier to identify the parameters of the model.
Also, with EDA, you can significantly improve the accuracy of the model even before it is built.
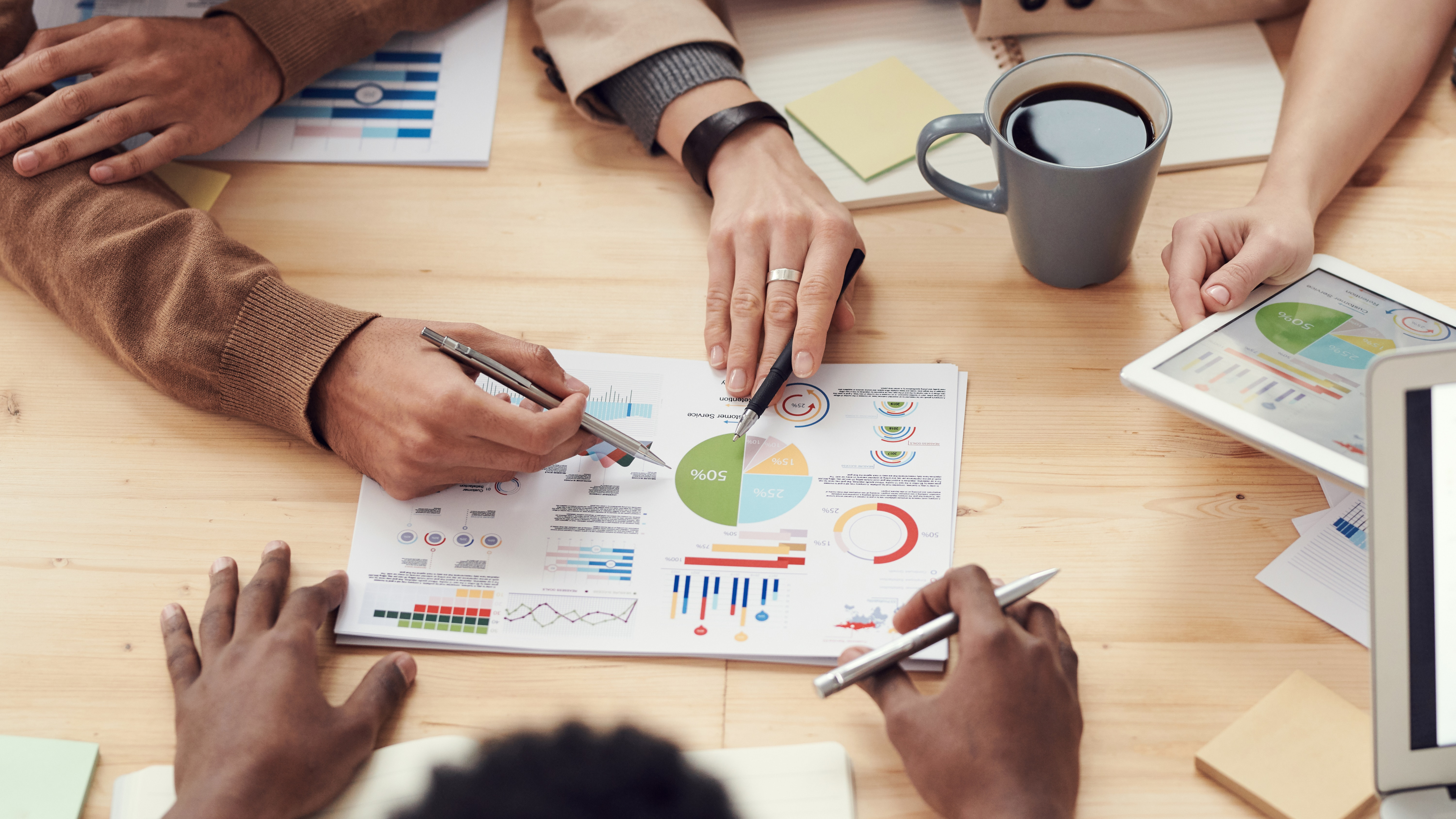
3- Building Predictive Models
Once exploratory data analysis is completed, it is time to start building the predictive models utilising machine learning (ML).
Predictor variables are used for making predictions on the target variables.
- Predictor – this is an independent attribute in the dataset that is used for predicting the target variable’s value. On the identification of the target, the remaining columns convert into predictor variables.
- Target – this is the dependent variable with unpredicted values.
Based on the problem you have in hand, you can either build a classification model or a regression model. You can use regression algorithms like multi-linear regression, simple linear regression, decision tree regression, and so on to obtain desired results. And these models come in handy when the target is a numeric feature. For instance, predicting the value of a home.
In case the target is in a categorical feature, classification models such as binary classification or multiclass classification are used.
Binary classification is done when the target has two possible categories. And when there are more than two possible outcome categories, multiclass classification is the reliable choice.
Based on the further requirement, even unsupervised algorithms like association and clustering algorithms can also be used.
Once the model is built, it is time to analyse the model’s performance.
To determine the most effective model, it is imperative to evaluate the model based on different parameters and scenarios. Typically, one or more metrics are utilized to identify how excellently the model performs.
The machine learning models are rated using different measuring systems. For instance, with the regression model, you can use Root Mean Squared Error (RMSE), Mean Squared Error (MSE) and R Squared (R2) score. With the classification model, Recall, Precision, AUC-ROC and Confusion Matrix are used.
4- Applying Predictive Analytics to Marketing
To grab the customer’s attention, marketers need to make a way through the ad noise and set their business apart.
As per Towards Data Science’s report, personalized messaging can help boost the customer experience, thereby improving the customer satisfaction rate. Through predictive analysis in marketing, it is possible to evaluate the customer’s cross-selling and upselling readiness.
You can upsell your customized services and products as per the customer’s preferences and cross-sell products related to them immediately.
With an in-depth knowledge of the customer base, you will know whether the campaign should be done through the company’s website, social media sites or emails.
Leading scoring can then be used for better marketing performance. As we know, an unqualified lead is only an unnecessary use of resources.
With the customer data generated through predictive analysis, it can become easy to determine the most profitable customers so you can nurture them and boost their chances of purchasing. With customer segmentation, you can optimize your marketing resources and set direction for your marketing campaigns.
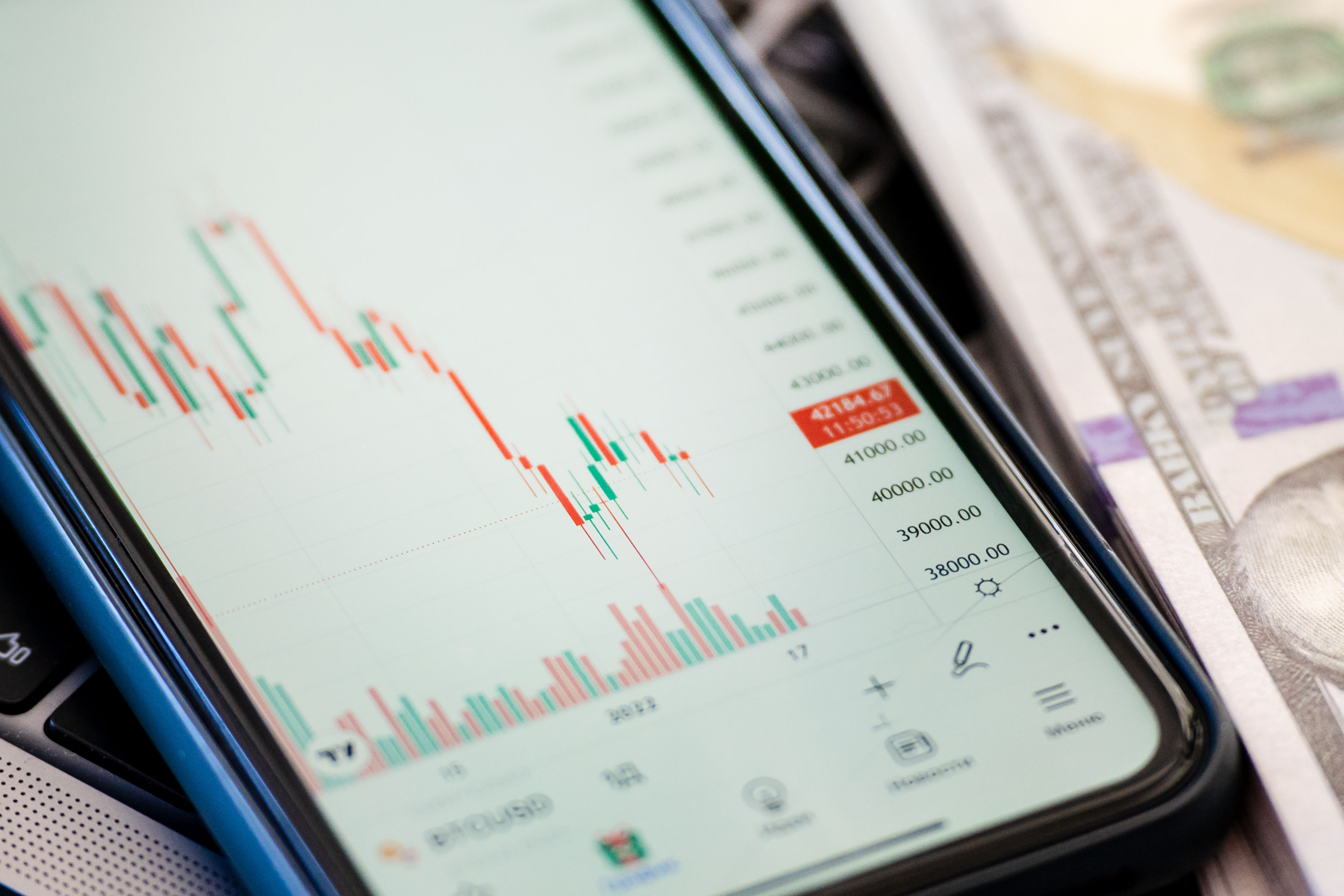
5. Measuring ROI of Predictive Analytics in Marketing
Measuring the success of predictive marketing analytics is essential to ensure the effectiveness of the process. Here are three key performance indicators (KPIs) for measuring predictive analytics success:
5.1 – Accuracy
The entire foundation of a predictive analysis model hinges on accuracy. This KPI measures how well the predictive model performs in predicting outcomes. It is calculated by comparing the predicted results with actual results. Here are some examples of KPIs for measuring accuracy:
- Precision: It measures the proportion of true positives (correctly predicted outcomes) to the total number of predicted outcomes. For example, a precision of 80% means that out of 100 predicted outcomes, 80 were correct.
- Recall: It keeps track of the proportion of true positives to the total number of actual outcomes (true positives + false negatives). So suppose the model shows a recall of 75%. This depicts that out of 100 actual outcomes, 75 were correctly predicted.
- Mean Absolute Error (MAE): It measures the average difference between the predicted and actual value. So, an MAE of 10 means that, on average, the predictions were off by 10 units.
5.2- ROI:
ROI (Return on Investment) is another measure of a well-performing predictive analysis model. Organizations track ROI, i.e., the value generated from predictive analytics in relation to the cost of implementing the process. Here are some examples of KPIs for measuring ROI:
- Cost per acquisition: It measures the cost of acquiring a new customer through predictive analytics. For example, if it costs $500 to acquire a new customer through predictive analytics, the cost per acquisition is $500. Hence, if the cost is high and the value derived by billing the customer is low, then it might turn out to be an unfeasible approach.
- Customer lifetime value (CLV): For many businesses, customer retention is way cheaper than customer acquisition. Hence it is important to keep track of this metric when monitoring the performance of the predictive analysis model. CLV measures the total value that a customer brings to the organization over their lifetime. Suppose a customer brings $10,000 in revenue over their lifetime. The CLV is $10,000. It is a good indicator of customer loyalty and low churn.
- Revenue increase: It measures the increase in revenue generated through predictive marketing analytics. Analysing past data and current trends and understanding customer preferences and viewpoints through the predictive analysis model will generate better revenues.
5.3- Speed
In today’s fast-paced business environment, speed is critical to stay competitive, in addition to the precision of data. Let us look at some KPIs for measuring speed:
- Response time: It measures the time taken by the predictive model to generate results. The lower the response time, the better the analytics model.
- Throughput: It measures the number of predictions the model can make in a given time. Shorter lead times from high-quality training datasets and suitable correlation analysis can ensure delivering immense value to a business in gleaning insights from the data. And it is worth tracking this KPI.
6- Best Practices for Implementing Predictive Analytics in Marketing
With significant amounts of data at their disposal, it becomes crucial for marketers to employ advanced marketing tools to take complete advantage of predictive analysis.
6.1- Unified marketing measurement
For prediction of the future trends, marketers require huge quantities of historical data and current data. This means that marketers must track everything from how consumers interact with the campaigns to when they move down the sales funnel and get converted.
All of these data then need to be synchronized and correlated to effectively generate consumer identities.
With unified marketing measurement, you can easily collect information on consumer behaviour, market trends, online and offline engagements, etc., in a centralized and seamless manner.
6.2- Marketing Analytics software
The integration between massive amounts of data and multiple measurement models is crucial for predictive analytics.
To take complete advantage of predictive marketing analytics, as a marketer, you need to keep advanced marketing software handy. Ensure your chosen software is capable of distilling all the data into comprehensible information that can assist in deriving actionable insights.
6.3- Artificial Intelligence and ML
With marketers leaning towards omnichannel marketing tools, AI and ML have become essential in marketing optimization. These tools assist marketers in taking action on the insights in real time, thereby automatically serving the dynamic content.
Depending upon predictive analytics, AI and ML marketing capabilities can provide automated sales forecasts, dynamic pricing, real-time personalization and automated content creation.
7- Case Studies
7.1- Airbnb
Did you know since its launch, AirBnB has grown by 43,000%? And most of this profit is due to their efforts and time spent on predictive analysis.
They employed a four-step process to create the predictive analytics system that resulted in the rapid growth –
- They took real time and historical data to produce theories and models about what might work and what might not.
- They created a plan to use the data generated in the most impactful way.
- They did A/B split tests and experiments to determine whether the finding was correct.
- They measured the data results and proceeded to repeat the process.
7.2- Amazon
Have you noticed how Amazon nails at suggesting “recommended items?” They are now deciding to take a step further with predictive ordering technology also known as anticipatory shipping.
So, what does this mean? People will now be able to order products they want even before purchasing them. Yes, you read it right.
Amazon’s predictive AI has become so advanced that now it will be able to determine what the customer wants to buy even before they buy it.
The anticipatory shipping will use Amazon’s astronomically huge database to identify what customers want beforehand. These items will then be shipped to the nearest hub to speed up the delivery time once the items actually get ordered.
The customers will get their items faster, enhancing the experience and chances of shopping more from Amazon.
7.3- Microsoft and Graemener
Would you have ever imagined using AI to preserve wildlife? Well, the Nisqually River Foundation partnered with Microsoft and Graemener and made that happen in reality.
Manually identifying and processing the fishes and their species in the 81-mile-long Nisqually River was nothing but a daunting task. Hence, Microsoft Azure and other similar tools were used to create AI models. Relevant data were fed into the AI model so it could do all the heavy lifting.
The outcome –
- The foundation was able to boost the accuracy of how the fishes were identified by 73%.
- It is also believed that the system given to the client will eventually assist with 80% cost-savings down the line.
It is evident from all three real-life use cases of predictive analytics that it works similarly to a crystal ball. It allows you to see into the future, thereby preventing any problems and finding lucrative opportunities.
8- Future of Predictive Analytics in Marketing
Even though predictive analytics has taken the business world by storm, it is worth noting that it is still a technology. And the best part is that this technology is developing at an accelerated rate.
As a marketer, business owner or analyst, you should stay prepared for what’s coming next.
Listed below are some predictions on how predictive analytics might develop in future –
- As of now, predictive analytics focuses on making predictions, tracking customer behaviour and so on. But, get ready for more specific applications that could result in greater diversification. For instance, you may come across software that assists companies in finding ways to generate more revenue. Software may also be developed for specific industries and niches like restaurants.
- Future apps and platforms may provide marketers with more control. This means you can create the advanced form of custom reports, integrations with other software and so on.
- Predictive analytics software will soon assist in projecting the data in a visual format. This way, you can gain intuitive takeaways and be able to easily communicate conclusions.
- Most predictive analytics software available in the market focus on high-level takeaways and broad-stroke insights. But in future, this software may dig deeper into a more individualized state. For instance, predicting the future behaviour of an individual customer or analysing past behaviours to assess the client’s current disposition.
Signing off
Improving the marketing ROI, customer retention and customer experience rate remain a few of the many priorities of marketers. To do so, they implement revolutionary techniques. Predictive analytics is one such technique. Although not a widespread choice yet, predictive analytics is slowly yet steadily gaining traction among marketers, too.
Predictive analytics, when used correctly, can result in overall business enhancement. From the application of sensor data to data point utilization for smarter data analytics, predictive models have countless benefits. Besides, the emerging trends in predictive analytics will only open various doors to drive marketing innovation.
Wait no more and leverage this innovation through marketing analytics software, unified marketing measurement and AI and machine learning to stay competitive in this data-driven market.